《Table 2 Parameters of multiple linear regression model (MLR) and geographically weighted regression
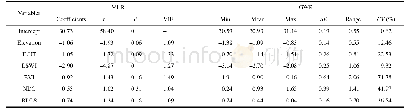
本系列图表出处文件名:随高清版一同展现
《Combining Environmental Factors and Lab VNIR Spectral Data to Predict SOM by Geospatial Techniques》
Notes:VIF:variance inflation factor;SE:standard error,CV:coefficient of variation;ILUT:the index of land use type,LSWI:land surface water index,EVI:enhanced vegetation index,NDC:the nearest distance to construction land,RDLS:roughness and relief ampli
PLSR and GWR were used to construct the prediction models of SOM by using the spectral reflectance,and the parameters are shown in Table 3.The first four PCs of the spectral reflectance were chosen as the auxiliary variables,since the accumulating contribution rate was80.85%and the eigenvalues were bigger than 1.The coefficients of intercept,PC1,PC2,PC3 and PC4,were30.73,3.49,2.90,2.92,and 2.26,respectively.PC1 had the biggest influence on SOM among all PCs.Because the datasets had been transformed,a p-value and VIF value equal to 0 and a t-value greater than 1 would suggest a statistically significant role of the PCs in the PLSR model.The PCs also had different influences on the SOM in different geographical locations based on the coefficients in GWR.The descending order of the mean values was PC1(3.57),PC3(3.01),PC2(2.92)and PC4(2.33).PC1 had the strongest influence on SOM,and PC4had the smallest influence.Meanwhile,coefficients of PC3had the greatest degree of variation(CV=10.61%),and coefficients of PC1 had the smallest variation(CV=5.10%).All of these PCs had a positive relationship with SOM since their coefficients were positive.
图表编号 | XD0046961900 严禁用于非法目的 |
---|---|
绘制时间 | 2019.04.01 |
作者 | GUO Long、ZHANG Haitao、CHEN Yiyun、QIAN Jing |
绘制单位 | College of Resources and Environment, Huazhong Agricultural University、College of Resources and Environment, Huazhong Agricultural University、School of Resource and Environment, Wuhan University、School of Resource and Environment, Wuhan University |
更多格式 | 高清、无水印(增值服务) |