《Table 3Performance of different classification strategies.》
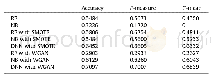
本系列图表出处文件名:随高清版一同展现
《基于Wasserstein GAN的新一代人工智能小样本数据增强方法——以生物领域癌症分期数据为例》
The RF and NB models trained with 4000 synthetic samples generated by WGAN exhibited different performance changes.The RF model had worse indexes than the model trained with the oversampling samples,while the NB model’s indexes increased considerably.These results imply that with the synthetic samples generated by WGAN,the classical machine-learning models did not provide good results.In the proposed framework,the deeplearning model DNN with synthetic samples from WGAN performed best among all the considered classifiers.Compared with the oversampling samples generated by SMOTE,this method further increased the accuracy from 64.52%to 70.97%,the F-measure value from 66.05%to 70.07%,and the G-mean value from63.32%to 68.39%,which demonstrates that WGAN with a DNN effectively solved the HCC stage recognition problem.Above all,these findings indicate that the deep-learning method can successfully be applied to a multi-classification problem with a limited number of samples.
图表编号 | XD0061019400 严禁用于非法目的 |
---|---|
绘制时间 | 2019.02.01 |
作者 | 刘宇飞、周源、刘欣、董放、王畅、王子鸿 |
绘制单位 | College of Life Science and Technology,Huazhong University of Science and Technology、School of Public Policy and Management,Tsinghua University、Center for Strategic Studies,Chinese Academy of Engineering、School of Public Policy and Management,Tsinghua Uni |
更多格式 | 高清、无水印(增值服务) |
查看“Table 3Performance of different classification strategies.”的人还看了
-
- Table 1 Comparison of different detection methods and strategies for evaluating PD-L1 expression levels