《Table 1 Parameter details of the proposed MSMLCNN》
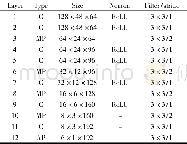
本系列图表出处文件名:随高清版一同展现
《Pedestrian attribute classification with multi-scale and multi-label convolutional neural networks》
First,it is very difficult to assign suitable weights to different attribute cost functions that are connected with different layers in the training progress.Second,attributes having complex localizing characteristics and different scales,the way only using features learned in the last layer is inappropriate,because the features learned in the last layer are too global to local attributes(e.g.,has sunglasses,upper Body VNeck and footwear Sandals).Therefore,as shown in Fig.2,in the proposed MSMLCNN,supervisory signals are added by fully connecting each attribute with multiple pooling layers at different scales,which makes the MSMLCNN able to learn multi-scale features for different attributes and apply multi-scale features for the testing phase.Parameter details of the proposed MSMLCNN is listed in Table 1.In the table C,MP and AP represent convolutional,max pooling and average pooling layers,respectively.
图表编号 | XD0020597200 严禁用于非法目的 |
---|---|
绘制时间 | 2018.03.01 |
作者 | 朱建清、Zeng Huanqiang、Zhang Yuzhao、Zheng Lixin、Cai Canhui |
绘制单位 | Fujian Academic Engineering Research Centre in Industrial Intellectual Techniques and Systems,College of Engineering,Huaqiao University、School of Information Science and Engineering,Huaqiao University、Fujian Academic Engineering Research Centre in Industr |
更多格式 | 高清、无水印(增值服务) |